Proposed topics for Masters thesis
(Scroll to the bottom of the page to check out some examples of past Masters theses)
Comparing observed and simulated snow line altitudes
The altitude of the snow line has significant implications for various fields such as water resources, ecology, and winter tourism. However, accurately predicting the snow line altitude remains a challenge. While remote sensing and webcam imagery can provide accurate observations, meteorological forecasts and reanalysis products struggle with estimating the zero-degree line, which is a strong predictor of the snow line altitude. Furthermore, whether precipitation falls as rain or snow depends on complex local phenomena such as humidity and temperature stratification, and whether snowfall sticks on the ground depends on surface temperature.
This project aims to evaluate the accuracy of meteorological products in estimating snow line altitude by comparing them to observed snow line altitude from webcam or remote sensing imagery. The resolution of meteorological products used for hydrological and ecological modeling is often insufficient for accurate snow line altitude modeling, and temperature estimates are also uncertain in complex terrain. By identifying systematic biases between observations and simulations, we may be able to apply bias-correction to meteorological product estimates, which eventually could improve the quality of hydrological and ecological models in mountainous areas.
Evaluation of ensemble precipitation products
In hydrology and snow models, rainfall predictions are often a major source of uncertainty. To tackle this, researchers use multiple model runs with slightly different rainfall predictions, creating what’s called an ensemble. This ensemble provides a range of results, from a narrow spread when the predictions are close, to a wide spread when the predictions vary a lot. Ideally, the actual outcome should fall somewhere within this range.
Currently, most studies generate these ensemble runs by taking a single rainfall prediction and making random adjustments to it. This helps account for uncertainty in the amount of rain but overlooks other important factors. For instance, it doesn’t consider uncertainties in where the rain will fall or whether there will be any rain at all. In hilly regions, like mountains, knowing the exact location of summer storms is tricky. If the prediction says a storm is in one catchment, it might actually hit a neighboring catchment. This uncertainty can have significant effects on streamflow. Unfortunately, this aspect of uncertainty is often ignored.
One way to tackle this is to use ensemble versions of common rainfall prediction models. While these models are usually available for free, their potential to create ensemble members for hydrology and snow modeling hasn’t been fully explored. This project aims to assess the quality of these ensemble rainfall predictions and their usefulness in creating ensemble members for hydrology and snow models in various Swiss regions with different characteristics.
Interpolation of speleothem-derived isoscapes for paleoclimate reconstruction
The isotopic composition of precipitation (mainly oxygen-18 and deuterium) provides valuable information on past temperatures, precipitation patterns, and atmospheric circulation. By analyzing isotopic records from various natural archives, we can reconstruct a picture of past climates, which is otherwise inaccessible. This can potentially inform our understanding of current and future climate change.
Speleothems (cave stalagmites) are a valuable record of precipitation isotopic composition. The SISAL database (https://pastglobalchanges.org/science/wg/sisal/intro) contains isotope data for hundreds of speleothems over the world, and spans thousands of years. This project will seek to interpolate this data in order to obtain worldwide isotope maps in the past (names isoscapes), using geostatistical and/or machine learning approaches.
References:
Erdélyi, D., Kern, Z., Nyitrai, T. et al. Predicting the spatial distribution of stable isotopes in precipitation using a machine learning approach: a comparative assessment of random forest variants. Int J Geomath 14, 14 (2023). https://doi.org/10.1007/s13137-023-00224-x
Dániel Erdélyi, István Gábor Hatvani, Hyeongseon Jeon, Matthew Jones, Jonathan Tyler, Zoltán Kern, Predicting spatial distribution of stable isotopes in precipitation by classical geostatistical- and machine learning methods, Journal of Hydrology, Volume 617, Part C, 2023, 129129, ISSN 0022-1694, https://doi.org/10.1016/j.jhydrol.2023.129129.
Mesure de précipitations par capteurs low-cost
La mesure des précipitations est typiquement faite avec des réseaux de mesure nationaux qui produisent des données de très bonne qualité, mais avec une couverture spatiale souvent peu dense, surtout en régions urbaines. Ce projet consiste à évaluer des capteurs de pluie très simples pouvant être distribués en un réseau dense sur une petite surface (p.ex. une ville), et à les comparer avec des capteurs classiques ou plus avancés tels que des pluviomètres ou des disdromètres.
Jaugeage de rivière par injection continue de traceur
Mesurer le débit d’une rivière est souvent difficile. La méthode classique consiste à construire une station de jaugeage, qui est un ouvrage de génie civil lourd. Une alternative intéressante est d’injecter un débit constant de traceur, et de mesurer la concentration en aval de façon continue. Cette méthode est bien moins coûteuse et beaucoup plus précise.
Ce projet consisterait à procéder à une série de jaugeages au traceur, et à les comparer à des mesures effectuées par station de gaugeage. Ceci comportera une composante de travail de terrain pour mettre au point et installer le mécanisme d’injection, et aussi pour installer un fluorimètre avec enregestrement continu pour mesurer la concentration de traceur.
Cartographie de la granulométrie des éboulis en haute montagne
Dans les régions de haute altitude, de grandes zones sont couvertes d’éboulis. La taille des grains qui composent ces éboulis est un facteur important contrôlant l’occurrence de permafrost et aussi l’apparence de végétation. Une méthode a été mise au point pour cartographier et identifier les grains à partir d’images prises par drones . Ce projet consiste à appliquer cette méthodologie sur une zone étendue des Alpes Vaudoises.
Estimation of driftwood accumulation in reservoirs using the Planet satellite imagery (together with Prof. Virginia Ruiz-Villanueva)
Instream wood (i.e., downed trees, trunks, rootwads and branches in rivers) is a key element of river ecosystems. Wood significantly affects river morphology and sediment dynamics, supporting biodiversity, influencing the nutrient and organic carbon cycles, and providing a variety of physical habitats in rivers. However, wood transport during floods may pose a risk because wood may block bridges and other infrastructures. Dams prevent the passage of instream wood to downstream reaches, and provide a depositional zone in which to quantify driftwood accumulation rates from upstream. Driftwood accumulations can be oberved in high resolution satellite images and therefore, quantified. The Planet constellation provides an unprecedented dataset of Earth observation imagery with a unique combination of coverage, frequency, and resolution. This dataset allows for the first time to quantify with a relatevely high temporal and spatial resolution the variation of the accumulations of driftwood in reservoirs.
This work will quantify the amount of driftwood that accumulates in a selected reservoir, using the Planet satellite imagery and analysing the wood amount variations with discharge and flood information and computing instream wood transport rates.
- Benacchio, V., Piégay, H., Buffin-Balanger, T., Vaudor, L., 2017. A new methodology for monitoring wood fluxes in rivers using a ground camera: Potential and limits. Geomorphology 279, 44–58. doi:10.1016/j.geomorph.2016.07.019
- Ruiz-Villanueva, V., Piégay, H., Gurnell, A.A., Marston, R.A., Stoffel, M., 2016. Recent advances quantifying the large wood dynamics in river basins: New methods and remaining challenges. Rev. Geophys. 54. doi:10.1002/2015RG000514
- Senter, A.E., Pasternack, G.B., Piégay, H., Vaughan, M.C., Lehyan, J.S., 2017. Wood export varies among decadal, annual, seasonal, and daily scale hydrologic regimes in a large, Mediterranean climate, mountain river watershed. Geomorphology 276. doi:10.1016/j.geomorph.2016.09.039
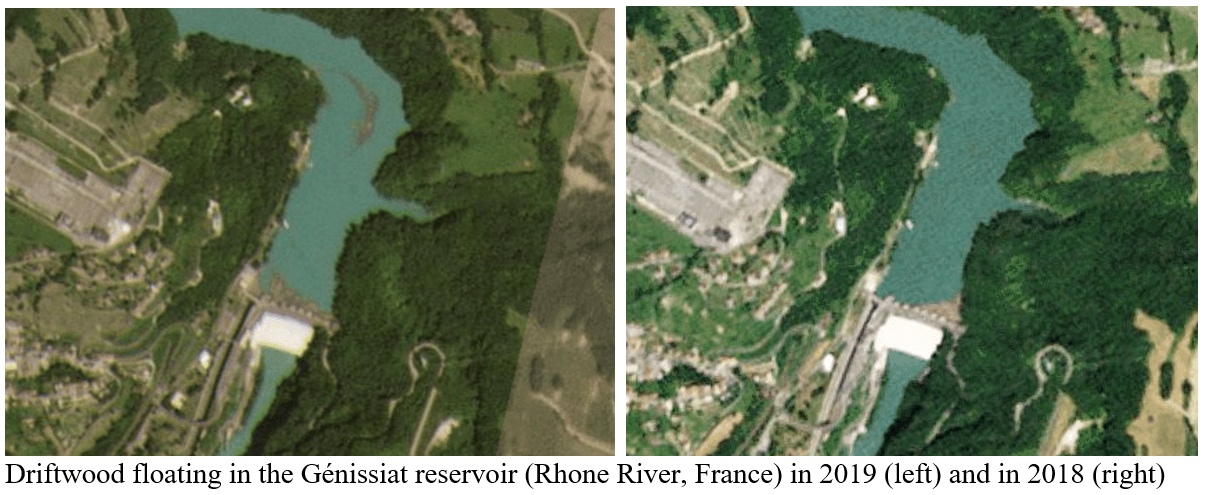
Downscaling of Digital Elevation Models with landscape evolution models
High-resolution Digital Elevation Models (DEMs) are often not available for studies of the earth surface. This project aims at testing an approach for downscaling DEMs using the laws governing erosion of a landscape (https://www.sciencedirect.com/science/article/pii/S0169555X12004618).
Etude de la relation entre pluviométrie et altitude à plusieurs échelles
La pluie est typiquement considérée comme corrélée à l’altitude, et cette relation est utilisée de façon générale pour interpoler des données ou pour formuler des modèles. Cependant, des données très locales semblent indiquer que cette relation n’est pas toujours valide lorsqu’on se focalise sur l’échelle de petits bassins-versants et sur des moyennes mensuelles ou saisonnières plutôt que annuelles. Il est possible que dans ces cas, d’autres facteurs peuvent être prépondérants pour expliquer l’intensité des pluies. Ce projet vise à établir de telles relations (ou absence de relations) en analysant des données de stations météorologiques dans les alpes.
Satellite image downscaling with block-Direct Sampling
A few years ago, a geostatistical simulation was developed to generate a stochastic image while respecting constraints imposed on a larger scale. This can potentially be used for downscaling. This topic will test the applicability of this idea. Here is the reference to the geostatistical simulation approach, for which a fully-functional code code is already available:
Straubhaar J, Renard P, Mariethoz G (2016). Conditioning multiple-point statistics simulations to block data. Spatial Statistics 16, 53-71, doi: 10.1016/j.spasta.2016.02.005.
Gap-filling of airborne hyperspectral imagery over Switzerland (AVIRIS-NG) using geostatistical approaches
An exceptional dataset of hyperspectral data is available over Switzerland. Unfortunately, the airborne survey covered only some parts of the country, leaving large gaps. This project involves applying geostatistical algorithms to determine whether it is possible to complete this dataset.
Quantify extreme temperature values on the earth surface using Landsat-8 TIRS
Landsat-8 has the highest-resolution long-term thermal sensor. It can allow to spot very local temperature extremes that are undetectable with lower resolution sensors. This project is to detect such extreme surface temperatures and possibly explain them.
Colorization and downscaling of drone imagery
Images acquired by drones often do not have many spectral bands (typically RGB). Multispectral camera can be mounted on drones, but then offer a lower resolution. This topic will apply a pansharpening method to add bands to drone images acquired with a high-resolution RGB camera, based on a lower resolution multispectral acquisition.
It will be based on an approach to statistically reconstruct the missing bands in images, described in this paper:
Gravey M, Rasera L, Mariethoz G (2019). Analogue-based colorization of remote sensing images using textural information, ISPRS Journal of Photogrammetry and Remote Sensing, 147: 242-254, doi: 10.1016/j.isprsjprs.2018.11.003.
Quantifying patterns of change in snow cover
Snow cover in alpine regions is complex. It is driven by many factors, such as altitude, slope, solar exposition, vegetation, among others. Daily satellite images allow observing the snowy areas and their evolution in great details. This project will use such data and morphological operators (https://en.wikipedia.org/wiki/Mathematical_morphology) to determine the processes through which snow appears and disappears throughout a season. Such methods allow defining the countours of the snow areas or their “patchiness”.
Simulation of future climatic time-series: preserving non-linear correlations and improving the delta-change approach with multiple-point statistics
Evaluating the future variability and trend of climatic variables is an important goal in climate-change research, involved in countless engineering, environmental and social applications. The delta-change approach is a widely used method to make an approximate estimation of local climate-change scenarios by simply scaling the available historical climate data by a given constant, issued from stochastic downscaling of climate models. However, the estimated variation is limited to certain climate variables, it does not take into account high-order statistical relations and the variability of different possible outcomes. The aim of this study is to explore the variability of a future delta-change scenario and its influence on multiple correlated variables by generating different stochastic realizations with a multiple-point stochastic simulation approach (MPS). MPS aims at generating realistic data patterns by considering the high-order statistical relations among different variables. The student will become familiar with the technique, test its application to climatic data and analyze the generated future climatic scenarios.
Merging radar and gauge measurements using multiple-point geostatistics
There are two main sources of data for operational measurement of rainfall: weather radars and rain gauges. While radar data are very useful to obtain spatial maps and to measure rain in inaccessible areas, it generally produces data that have a relatively low spatial resolution and that present numerous biases. Rain gauges data are generally much more accurate, but only available as point measurements, which need to be interpolated, thus resulting in significant uncertainty. Several methods exist to merge these two sources of data, however they often involve assumptions that result in a misrepresentation of rainfall heterogeneity. Moreover, the final product often does not match what is measured in the rain gauges (e.g. the radar indicates rain where rain gauges measured no rain).
This project will investigate the use of multiple-point geostatistics methods, to generate rain fields that are consistent with the heterogeneity of the radar data, and that are in agreement with the rain gauges. It will involve using existing software and available MeteoSuisse data products.
Comparison of methods for clouds removal on satellite images
Increasingly, high-frequency images of virtually every place on earth are available to the scientific community. However, the presence of clouds on satellite images is one of the main obstacles that prevent us from having data available everywhere and all the time. Many methods have been proposed to reconstruct the missing parts of the images.
This work will focus on the rather common situation, where we have a set of images for a certain region, acquired on different dates. Some images are affected by the clouds, others are not, and we can reconstruct the surface information by cross-checking the information of both images. The goal will be, from satellite data acquired in different environments, to test different methods proposed in the literature and to conduct a quantitative comparison.
Jaugeage de grands fleuves par satellite
Connaître le débit des cours d’eau est essentiel pour pouvoir comprendre le fonctionnement hydrologique d’un bassin-versant, pour prédire les événements tels que les crûes et pour quantifier l’impact de l’activité humaine sur l’environnement. Cependant, effectuer des mesures de débit à intervalles réguliers est difficile et cher, car nécessitant l’installation de stations de jaugeage qui représentent de lourds investissements.
Ce projet vise à investiguer l’utilisation d’images prises par télédétection pour mesurer le débit des rivières. Ceci serait en particulier utile dans les bassins-versants non-jaugés des pays en développement, qui n’ont pas les moyens d’installer des stations de jaugeage. L’idée serait d’utiliser des images satellite ou aériennes pour déterminer la surface inondée. Ensuite, un modèle numérique de terrain précis peut permettre de déduire la pente de la rivière ainsi que le profil de la rivière sur un certain nombre de sections. Un autre paramètre important est le coefficient de friction qui dépend de la forme du lit de la rivière. En utilisant ces éléments et certaines lois de mécanique des fluides, il devrait être possible d’estimer le début d’une rivière.
Ce projet consistera à tester la faisabilité d’une telle méthode.
Simulating time series with extreme values using multiple-point geostatistics
In recent years, resampling-based approaches have emerged as a useful way of generating stochastic time series. They allow predicting the characteristics of future rainfall or streamflow considering a range of complex behaviors, which is difficult to accomplish with other approaches. Moreover, resampling-based methods are easy to understand and to implement, and therefore attractive from an application point of view. However, one limitation of those approaches is the simulation of extreme values. Indeed, when resampling training data, it is not possible to generate higher values than those already available in the training data.
This project will explore one possible workaround to generate extreme values in the context of resampling approaches, using the framework of multiple-point geostatistics. Time series generation usually involves using as input a training dataset that has a given temporal resolution (e.g. hourly) and simulate at the same resolution. The idea explored here is to first downscale the training data (e.g. a half-hourly resolution), and use this downscaled data for training. The resulting simulation (half-hourly) is then upscaled again (to an hourly resolution). This procedure can generate values outside the range of the training data, and can be repeated iteratively to enhance the extreme values. The downscaling approach used should also play a role in the magnitude of the extremes.
This project will test the approach using existing resampling-based codes that are developed in the GAIA group.
Simulating storms through stochastic simulation of space-time convective fields using multiple-point geostatistics
The detailed observation of rainfall storms in space and time is of primary importance to evaluate the risks and uncertainty related to urban systems. Last-generation X-band radars can acquire high-resolution images of rainfall convection in order to study its behavior and impact on infrastructures. Unfortunately, such data are still very expensive and rarely available, but stochastic simulation techniques may be used to generate synthetic fields honoring the same spatiotemporal properties. The aim of this project is to elaborate a specific simulation framework based on multiple-point statistics (MPS), a methodology based on the random generation of complex data patterns as observed in the available data. The student will become familiar with the technique and test its application to the generation of space-time rainfall fields using radar images as training data. The aim will be to preserve realistic properties of convective rainfall and possibly use auxiliary data such as satellite images to focus the study on specific rainfall events.
Investigating the influence of shadows on reflectance spectra
When using remote sensing in mountain areas, shadows can pose significant problems. For a given surface type, the reflectance spectrum can be very different depending on the shadow/sunlit condition. In shadow areas, most of the incident light comes from reflection on surrounding slopes, as well as from skylight (atmospheric effects). As a result, classification of corresponding images can be challenging and often one has to define separate classes for shadown and sunlit areas.
This project will investigate ways to relate the reflectance spectra of shadowy and sunlit areas, for a given surface type. Snow surfaces are of particular interest because the high reflectance implies that surrounding slopes will have a strong influence on shadow areas. The work will involve a comprehensive literature review, followed by a systematic comparison of spectra acquired in mountain regions, for images coming from different satellite platforms, including Sentinel-2, Landsat and MODIS.
Some past masters theses
Jason Bula (2019) – PDF
Dionys Lugon-Moulin (2019) – PDF
Myriam Eggerschwiler (2018) – PDF
Rachel Bungener (2018) – PDF
Danick Perrin (2018) – PDF
Raphael Vallat (2018) – PDF
Romain Udry (2017) – PDF