Proposed topics for Masters thesis
(Scroll to the bottom of the page to check out some examples of past Masters theses)
Deep learning for streamflow-based snow mass reconstructions
Deep learning models have been applied to many different areas of hydrology over the last several years, with a lot of success. One area where they have not yet been applied is in the reconstruction of 20th century snow mass evolution. In the 21st century there have been many snow mass reconstructions using remote sensing and snow station observations, but in the 20th century there are many years in many catchments where we have streamflow observations and meteorological observations, but still no snow mass reconstructions. In this project, you will apply a deep learning model to learn the relationship between streamflow, meterological observations and 21st century snow mass reconstructions. The model takes streamflow and meteorological observations as input and produces snow mass as output. After training on 21st century data, we can apply the model on the 20th century and obtain the snow mass reconstructions that are currently still missing. Retrieving this historical snow information could tell us more about the impact of climate change on the amount of snow in our mountains and in our rivers.
Reconstructing snow accumulation in 100+ catchments using precipitation and streamflow
Throughout the winter in mountainous catchments, we measure precipitation without having discharge, whereas in spring we have discharge without measuring precipitation. The deficit between the accumulated precipitation and the accumulated discharge gives us an estimate of the total amount of snowfall over the course of one winter. This technique of measuring snow accumulation is called the mass-curve technique, and in this project you will apply it to 100+ catchments to discover variability and trends in snow accumulation over the last 50+ years.
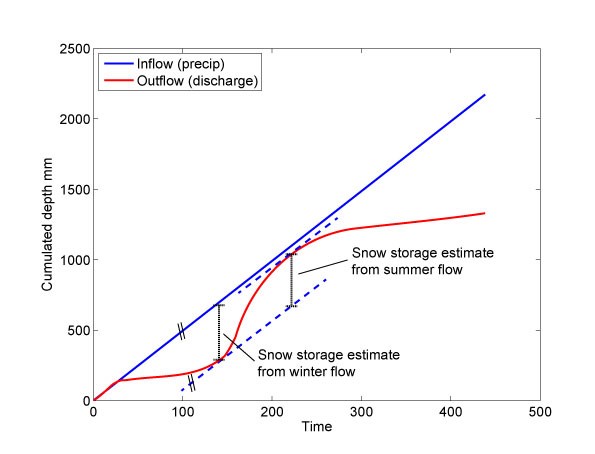
Machine learning-based hydrograph seperation
Streamflow time series of mountainous catchments are a mix of rainfall and snowfall output. It is difficult to unmix them, to estimate the partition between rainfall and snowfall just from looking at the discharge. In this project, you will give it a try with a machine learning algorithm. The alrogithm will be trained in an artificial setting, where we know both the inputs (mixed rainfall/snowfall discharge) and the outputs (unmixed rainfall+snowfall partitions). Once it works on the artifical setting, you can try it out on real discharge time series.
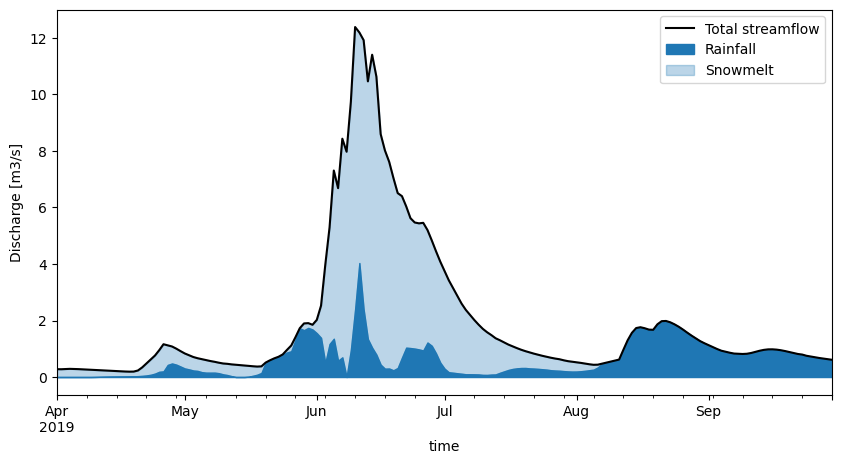
Comparing observed and simulated snow line altitudes
The snow line altitude is crucial for water resources, ecology, and winter tourism, but predicting it accurately is challenging. While remote sensing and webcam imagery provide reliable observations, meteorological forecasts and reanalysis products often struggle to estimate the zero-degree line, a key predictor of the snow line altitude. Local factors such as humidity, temperature stratification, and surface conditions further complicate the prediction of whether precipitation falls as snow or rain.
This project will compare snow line altitudes estimated by meteorological products with observations from webcams and remote sensing imagery. Given the limitations in resolution and temperature accuracy in mountainous terrain, identifying systematic biases in these products could help apply corrections, ultimately improving hydrological and ecological models in these regions.
Interpolation of speleothem-derived isoscapes for paleoclimate reconstruction
The isotopic composition of precipitation (mainly oxygen-18 and deuterium) provides valuable information on past temperatures, precipitation patterns, and atmospheric circulation. By analyzing isotopic records from various natural archives, we can reconstruct a picture of past climates, which is otherwise inaccessible. This can potentially inform our understanding of current and future climate change.
Speleothems (cave stalagmites) are a valuable record of precipitation isotopic composition. The SISAL database (https://pastglobalchanges.org/science/wg/sisal/intro) contains isotope data for hundreds of speleothems over the world, and spans thousands of years. This project will seek to interpolate this data in order to obtain worldwide isotope maps in the past (names isoscapes), using geostatistical and/or machine learning approaches.
References:
Erdélyi, D., Kern, Z., Nyitrai, T. et al. Predicting the spatial distribution of stable isotopes in precipitation using a machine learning approach: a comparative assessment of random forest variants. Int J Geomath 14, 14 (2023). https://doi.org/10.1007/s13137-023-00224-x
Dániel Erdélyi, István Gábor Hatvani, Hyeongseon Jeon, Matthew Jones, Jonathan Tyler, Zoltán Kern, Predicting spatial distribution of stable isotopes in precipitation by classical geostatistical- and machine learning methods, Journal of Hydrology, Volume 617, Part C, 2023, 129129, ISSN 0022-1694, https://doi.org/10.1016/j.jhydrol.2023.129129.
Jaugeage de rivière par injection continue de traceur
Mesurer le débit d’une rivière est souvent difficile. La méthode classique consiste à construire une station de jaugeage, qui est un ouvrage de génie civil lourd. Une alternative intéressante est d’injecter un débit constant de traceur, et de mesurer la concentration en aval de façon continue. Cette méthode est bien moins coûteuse et beaucoup plus précise.
Ce projet consisterait à procéder à une série de jaugeages au traceur, et à les comparer à des mesures effectuées par station de gaugeage. Ceci comportera une composante de travail de terrain pour mettre au point et installer le mécanisme d’injection, et aussi pour installer un fluorimètre avec enregestrement continu pour mesurer la concentration de traceur.
Cartographie de la granulométrie des éboulis en haute montagne
Dans les régions de haute altitude, de grandes zones sont couvertes d’éboulis. La taille des grains qui composent ces éboulis est un facteur important contrôlant l’occurrence de permafrost et aussi l’apparence de végétation. Une méthode a été mise au point pour cartographier et identifier les grains à partir d’images prises par drones . Ce projet consiste à appliquer cette méthodologie sur une zone étendue des Alpes Vaudoises.
Analyse de séries de débits dans le Val d’Heremence
Ce sujet consistera à analyser de longues séries temporelles de début dans la région du barrage de la Grande Dixence. A partir de données historiques de débit, il est possible de déduire la durée et l’intensité des périodes de fonte de neige, et partant d’estimer les conditions d’enneigement à des époques lointaines dans le passé (i.e. lorsque les données satellite n’étaient pas encore disponibles).
Reconstruction de données d’évaporation synthétiques pour le Bassin de la volta
Dans la région du bassin de la Volta (Afrique de l’Ouest), des millions de personnes dépendent de ressources en eau dont le régime est peu informé en données. Ce sujet consistera à mettre en oeivre des méthodes statistiques développées dans notre groupe pour générer des données d’évapotranspiration synthétiques mais plausibles, pour des périodes où les données satellite de sont pas disponibles (p.ex. dans le passé). Le but final est de pouvoir reconstruire l’état du bassin-versant sur une longue période à l’aide de modèles qui ont besoin de ce genre de données.
Estimation of driftwood accumulation in reservoirs using the Planet satellite imagery (together with Prof. Virginia Ruiz-Villanueva)
Instream wood (i.e., downed trees, trunks, rootwads and branches in rivers) is a key element of river ecosystems. Wood significantly affects river morphology and sediment dynamics, supporting biodiversity, influencing the nutrient and organic carbon cycles, and providing a variety of physical habitats in rivers. However, wood transport during floods may pose a risk because wood may block bridges and other infrastructures. Dams prevent the passage of instream wood to downstream reaches, and provide a depositional zone in which to quantify driftwood accumulation rates from upstream. Driftwood accumulations can be oberved in high resolution satellite images and therefore, quantified. The Planet constellation provides an unprecedented dataset of Earth observation imagery with a unique combination of coverage, frequency, and resolution. This dataset allows for the first time to quantify with a relatevely high temporal and spatial resolution the variation of the accumulations of driftwood in reservoirs.
This work will quantify the amount of driftwood that accumulates in a selected reservoir, using the Planet satellite imagery and analysing the wood amount variations with discharge and flood information and computing instream wood transport rates.
- Benacchio, V., Piégay, H., Buffin-Balanger, T., Vaudor, L., 2017. A new methodology for monitoring wood fluxes in rivers using a ground camera: Potential and limits. Geomorphology 279, 44–58. doi:10.1016/j.geomorph.2016.07.019
- Ruiz-Villanueva, V., Piégay, H., Gurnell, A.A., Marston, R.A., Stoffel, M., 2016. Recent advances quantifying the large wood dynamics in river basins: New methods and remaining challenges. Rev. Geophys. 54. doi:10.1002/2015RG000514
- Senter, A.E., Pasternack, G.B., Piégay, H., Vaughan, M.C., Lehyan, J.S., 2017. Wood export varies among decadal, annual, seasonal, and daily scale hydrologic regimes in a large, Mediterranean climate, mountain river watershed. Geomorphology 276. doi:10.1016/j.geomorph.2016.09.039
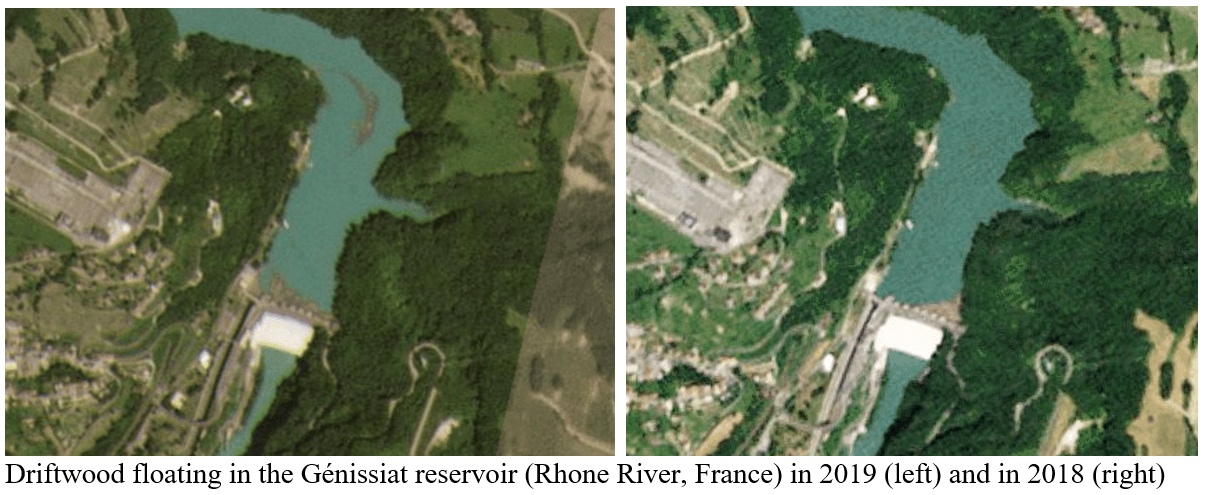
Downscaling of Digital Elevation Models with landscape evolution models
High-resolution Digital Elevation Models (DEMs) are often not available for studies of the earth surface. This project aims at testing an approach for downscaling DEMs using the laws governing erosion of a landscape (https://www.sciencedirect.com/science/article/pii/S0169555X12004618).
Etude de la relation entre pluviométrie et altitude à plusieurs échelles
La pluie est typiquement considérée comme corrélée à l’altitude, et cette relation est utilisée de façon générale pour interpoler des données ou pour formuler des modèles. Cependant, des données très locales semblent indiquer que cette relation n’est pas toujours valide lorsqu’on se focalise sur l’échelle de petits bassins-versants et sur des moyennes mensuelles ou saisonnières plutôt que annuelles. Il est possible que dans ces cas, d’autres facteurs peuvent être prépondérants pour expliquer l’intensité des pluies. Ce projet vise à établir de telles relations (ou absence de relations) en analysant des données de stations météorologiques dans les alpes.
Satellite image downscaling with block-Direct Sampling
A few years ago, a geostatistical simulation was developed to generate a stochastic image while respecting constraints imposed on a larger scale. This can potentially be used for downscaling. This topic will test the applicability of this idea. Here is the reference to the geostatistical simulation approach, for which a fully-functional code code is already available:
Straubhaar J, Renard P, Mariethoz G (2016). Conditioning multiple-point statistics simulations to block data. Spatial Statistics 16, 53-71, doi: 10.1016/j.spasta.2016.02.005.
Quantify extreme temperature values on the earth surface using Landsat-8 TIRS
Landsat-8 has the highest-resolution long-term thermal sensor. It can allow to spot very local temperature extremes that are undetectable with lower resolution sensors. This project is to detect such extreme surface temperatures and possibly explain them.
Colorization and downscaling of drone imagery
Images acquired by drones often do not have many spectral bands (typically RGB). Multispectral camera can be mounted on drones, but then offer a lower resolution. This topic will apply a pansharpening method to add bands to drone images acquired with a high-resolution RGB camera, based on a lower resolution multispectral acquisition.
It will be based on an approach to statistically reconstruct the missing bands in images, described in this paper:
Gravey M, Rasera L, Mariethoz G (2019). Analogue-based colorization of remote sensing images using textural information, ISPRS Journal of Photogrammetry and Remote Sensing, 147: 242-254, doi: 10.1016/j.isprsjprs.2018.11.003.
Quantifying patterns of change in snow cover
Snow cover in alpine regions is complex. It is driven by many factors, such as altitude, slope, solar exposition, vegetation, among others. Daily satellite images allow observing the snowy areas and their evolution in great details. This project will use such data and morphological operators (https://en.wikipedia.org/wiki/Mathematical_morphology) to determine the processes through which snow appears and disappears throughout a season. Such methods allow defining the countours of the snow areas or their “patchiness”.
Merging radar and gauge measurements using multiple-point geostatistics
There are two main sources of data for operational measurement of rainfall: weather radars and rain gauges. While radar data are very useful to obtain spatial maps and to measure rain in inaccessible areas, it generally produces data that have a relatively low spatial resolution and that present numerous biases. Rain gauges data are generally much more accurate, but only available as point measurements, which need to be interpolated, thus resulting in significant uncertainty. Several methods exist to merge these two sources of data, however they often involve assumptions that result in a misrepresentation of rainfall heterogeneity. Moreover, the final product often does not match what is measured in the rain gauges (e.g. the radar indicates rain where rain gauges measured no rain).
This project will investigate the use of multiple-point geostatistics methods, to generate rain fields that are consistent with the heterogeneity of the radar data, and that are in agreement with the rain gauges. It will involve using existing software and available MeteoSuisse data products.
Comparison of methods for clouds removal on satellite images
Increasingly, high-frequency images of virtually every place on earth are available to the scientific community. However, the presence of clouds on satellite images is one of the main obstacles that prevent us from having data available everywhere and all the time. Many methods have been proposed to reconstruct the missing parts of the images.
This work will focus on the rather common situation, where we have a set of images for a certain region, acquired on different dates. Some images are affected by the clouds, others are not, and we can reconstruct the surface information by cross-checking the information of both images. The goal will be, from satellite data acquired in different environments, to test different methods proposed in the literature and to conduct a quantitative comparison.
Jaugeage de grands fleuves par satellite
Connaître le débit des cours d’eau est essentiel pour pouvoir comprendre le fonctionnement hydrologique d’un bassin-versant, pour prédire les événements tels que les crûes et pour quantifier l’impact de l’activité humaine sur l’environnement. Cependant, effectuer des mesures de débit à intervalles réguliers est difficile et cher, car nécessitant l’installation de stations de jaugeage qui représentent de lourds investissements.
Ce projet vise à investiguer l’utilisation d’images prises par télédétection pour mesurer le débit des rivières. Ceci serait en particulier utile dans les bassins-versants non-jaugés des pays en développement, qui n’ont pas les moyens d’installer des stations de jaugeage. L’idée serait d’utiliser des images satellite ou aériennes pour déterminer la surface inondée. Ensuite, un modèle numérique de terrain précis peut permettre de déduire la pente de la rivière ainsi que le profil de la rivière sur un certain nombre de sections. Un autre paramètre important est le coefficient de friction qui dépend de la forme du lit de la rivière. En utilisant ces éléments et certaines lois de mécanique des fluides, il devrait être possible d’estimer le début d’une rivière.
Ce projet consistera à tester la faisabilité d’une telle méthode.
Simulating time series with extreme values using multiple-point geostatistics
In recent years, resampling-based approaches have emerged as a useful way of generating stochastic time series. They allow predicting the characteristics of future rainfall or streamflow considering a range of complex behaviors, which is difficult to accomplish with other approaches. Moreover, resampling-based methods are easy to understand and to implement, and therefore attractive from an application point of view. However, one limitation of those approaches is the simulation of extreme values. Indeed, when resampling training data, it is not possible to generate higher values than those already available in the training data.
This project will explore one possible workaround to generate extreme values in the context of resampling approaches, using the framework of multiple-point geostatistics. Time series generation usually involves using as input a training dataset that has a given temporal resolution (e.g. hourly) and simulate at the same resolution. The idea explored here is to first downscale the training data (e.g. a half-hourly resolution), and use this downscaled data for training. The resulting simulation (half-hourly) is then upscaled again (to an hourly resolution). This procedure can generate values outside the range of the training data, and can be repeated iteratively to enhance the extreme values. The downscaling approach used should also play a role in the magnitude of the extremes.
This project will test the approach using existing resampling-based codes that are developed in the GAIA group.
Investigating the influence of shadows on reflectance spectra
When using remote sensing in mountain areas, shadows can pose significant problems. For a given surface type, the reflectance spectrum can be very different depending on the shadow/sunlit condition. In shadow areas, most of the incident light comes from reflection on surrounding slopes, as well as from skylight (atmospheric effects). As a result, classification of corresponding images can be challenging and often one has to define separate classes for shadown and sunlit areas.
This project will investigate ways to relate the reflectance spectra of shadowy and sunlit areas, for a given surface type. Snow surfaces are of particular interest because the high reflectance implies that surrounding slopes will have a strong influence on shadow areas. The work will involve a comprehensive literature review, followed by a systematic comparison of spectra acquired in mountain regions, for images coming from different satellite platforms, including Sentinel-2, Landsat and MODIS.
Some past masters theses
Candice Dubath (2023) – PDF
Jason Bula (2019) – PDF
Dionys Lugon-Moulin (2019) – PDF
Myriam Eggerschwiler (2018) – PDF
Rachel Bungener (2018) – PDF
Danick Perrin (2018) – PDF
Raphael Vallat (2018) – PDF
Romain Udry (2017) – PDF