Contact: said.obakrim@unil.ch
Github: https://github.com/sobakrim
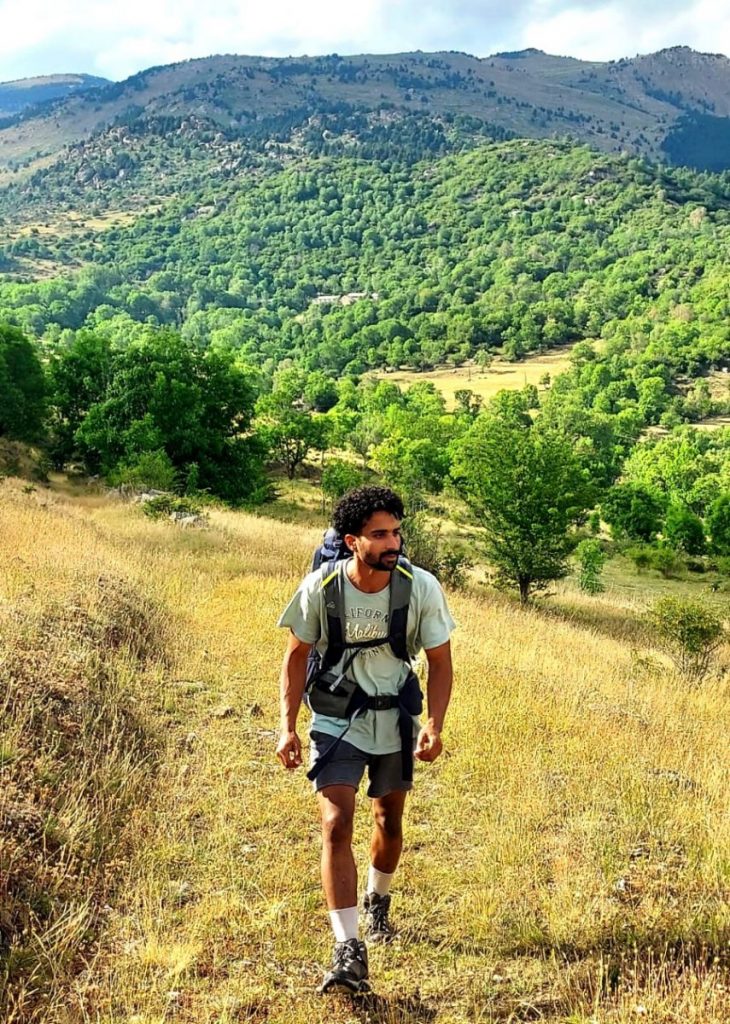
Current position:
I am a postdoctoral researcher in the Geostatistical Algorithms & Image Analysis (GAIA) research group at the University of Lausanne. My postdoctoral work focuses on the generation of synthetic images with geostatistical models and the development of new metrics to validate the space-time statistics of the generated images.
Research Interests:
My main research area encompasses statistics, space-time statistics, and machine learning, with a particular emphasis on applications in climate and environmental science.
Former positions:
From 2022 to 2024 I did my first postdoc at Biostatistics and Spatial Processes (BioSP) unit, at INRAE Avignon, where I worked on the development of a multivariate stochastic space-time weather generator using the latest developments in space-time statistics.
From 2019 to 2022 I did my PhD at Institut de Recherche Mathématique de Rennes (IRMAR) and Ifremer at Brest where I was based. The main objective of my PhD was to create statistical models for predicting ocean wave heights using wind conditions.
Publications:
Obakrim, S., Benoit, L., Allard, D., A multivariate and space-time stochastic weather generator using a latent Gaussian framework. (2024). https://hal.science/hal-04715860
Alglave, B., Dufée, B., Obakrim, S., James T Thorson. Empirical Orthogonal Functions for ecology. 2024. https://hal.science/hal-04693871/
Obakrim, S., Ailliot, P., Monbet, V., & Raillard, N. (2024). EM algorithm for generalized Ridge regression with spatial covariates. Environmetrics, e2871. https://doi.org/10.1002/env.2871
Obakrim, S., Ailliot, P., Monbet, V., & Raillard, N. (2023). Statistical modeling of the space–time relation between wind and significant wave height. Advances in Statistical Climatology, Meteorology and Oceanography, 9(1), 67-81. https://doi.org/10.5194/ascmo-9-67-2023
Obakrim, S., Monbet, V., Raillard, N., & Ailliot, P. (2023). Learning the spatiotemporal relationship between wind and significant wave height using deep learning. Environmental Data Science, 2, e5. https://doi.org/10.1017/eds.2022.35
Michel M, Obakrim S, Raillard N, Ailliot P, Monbet V. Deep learning for statistical downscaling of sea states. Advances in Statistical Climatology, Meteorology and Oceanography. 2022 Apr 7;8(1):83-95. https://doi.org/10.5194/ascmo-8-83-2022