Cette publication est également disponible en :
Français
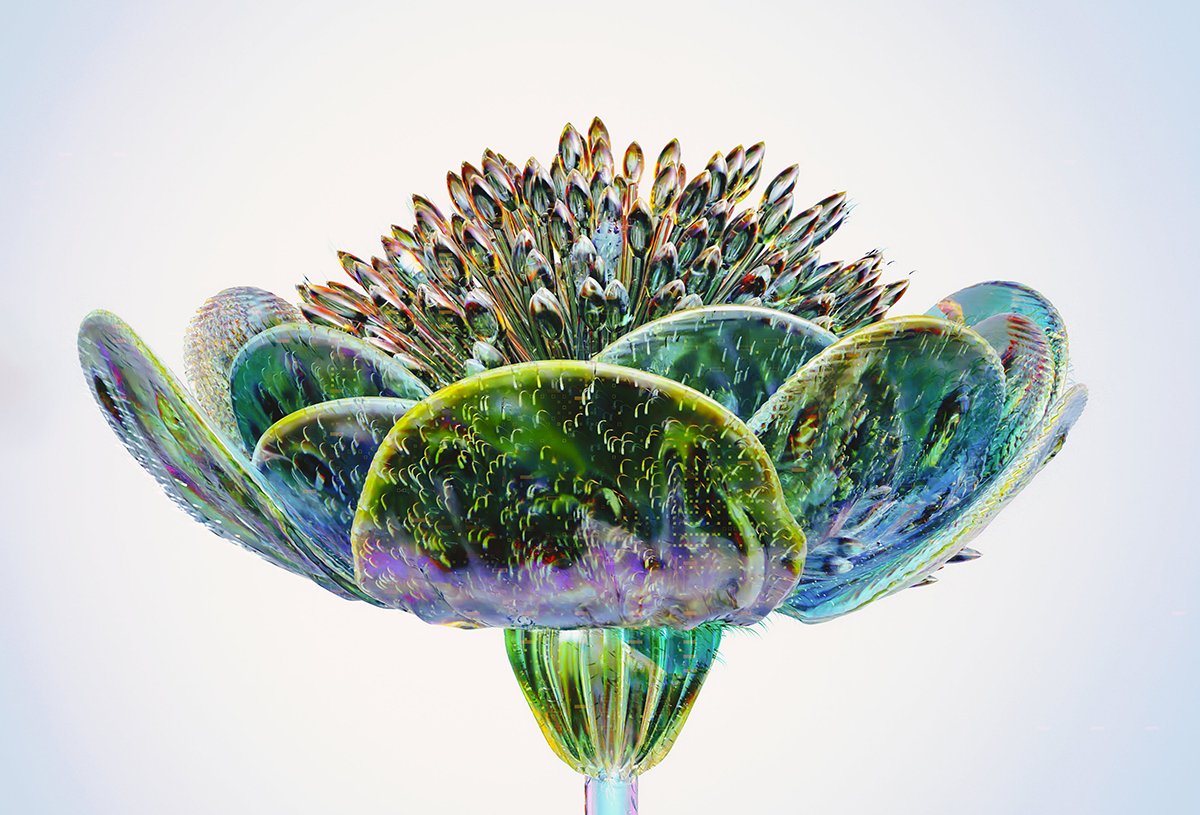
Machine learning algorithms, which are increasingly used in climate applications, are currently faced with a major problem: their difficulty in correctly predicting climate regimes for which they are not trained, thus generating uncertainties in projections. In a study published in the journal Science Advances, a team of researchers from the University of Lausanne and several American universities have revealed that, by transforming the data submitted to the algorithms using well-established physics principles, they can make them more “robust” for solving climate problems. This method has been successfully tested on three different atmospheric models. The implications of this finding go beyond climate science.
Climate change projection is a generalization based on extrapolation from the recent past using physical models for past, present and future climates. However, current climate models face challenges related to the need to represent processes at scales smaller than the model grid size, thus generating uncertainties in projections. Although recent machine learning algorithms offer advantages for improving these process representations, they show a tendency to extrapolate poorly to climate regimes for which they are not trained.
To overcome these limitations, the research team has proposed an innovative approach called “Climate-invariant Machine Learning”. This approach seeks to merge the physical understanding of climate models with the capabilities of machine learning algorithms to improve consistency, data efficiency, and generalization across diverse climate regimes. The results suggest that this integration of physical knowledge could strengthen the reliability of data-driven climate process models in the future.
Seven points to better understand the key aspects of the study:
1. What climate applications are machine learning algorithms used for?
Machine learning (ML) algorithms play a key role in enhancing climate models by simulating intricate processes such as storm dynamics, oceanic eddies, and cloud formation, which are costly with traditional methods. They’re pivotal in remote sensing for cloud detection and classification, and for downscaling global climate models to produce detailed local projections that better align with our observational record.
2. Why have machine learning algorithms struggled with predicting climate change effects?
Machine learning models, particularly neural networks, excel within the scope of their training data but can falter significantly with data that differ markedly from what they’ve seen before. This discrepancy arises because these models make implicit assumptions that may not hold true under novel climate conditions, leading to potential inaccuracies in projections outside their training regimes.
3. What atmospheric models did the study focus on?
The initial focus was on an “ocean world” model, a simplified representation of Earth’s climate system without continents, which helped identify and understand errors in extrapolation. The researchers progressed to more sophisticated, realistic atmospheric models that simulate the Earth’s climate dynamics. Integrating machine learning into these models is a promising venture to improve their realism, particularly for long-term climate projections, thereby contributing significantly to climate change adaptation and mitigation strategies.
4. What does this mean beyond climate science?
Beyond climate science, the methodology of the study offers a blueprint for incorporating physical principles into machine learning across various disciplines. By transforming the data using known physical invariances, the researchers can train ML models that work across various physical regimes despite only having been trained in a couple of them. This could work in any scientific domain with known invariances, e.g., in planetary science to create models generalizing across planets or in fluid dynamics to create models generalizing across flow regimes.
5. How could this change climate science research?
The study’s approach has the potential to advance climate science by enabling more accurate and generalizable process modeling. For example, machine learning models that have been trained on current climate data could, with appropriate physical adjustments, offer reliable projections for future climates. This breakthrough in generalization could lead to advancements in both weather forecasting and long-term climate projections.
6. What are the next steps for this research?
The groups which participated in this collaboration are exploring diverse avenues, including enhancing the generalizability of state-of-the-art data-driven weather forecasting models to future climates and incorporating these robust, climate-invariant modules into existing climate models. The goal is to keep pushing these data-driven models beyond their current limits, fostering a culture of rigorous and vigorous testing that could unearth new physical principles that extend beyond current observational capabilities.
7. What’s the long-term impact of these findings and the potential of AI in climate science?
The authors of the study anticipate these findings will foster deeper collaborations between the climate science and artificial intelligence communities. By encouraging the climate science community to view data-driven models not as a replacement but as an augmentation to traditional methodologies, and by promoting the development of AI techniques that are not just data-informed but also domain-aware, they anticipate a future where AI contributes significantly to advancing our understanding of climate processes, thereby enhancing collective ability to respond to the challenges posed by climate change.
Additional information
Tom Beucler, Pierre Gentine, Janni Yuval, Ankitesh Gupta, Liran Peng, Jerry Lin, Sungduk Yu, Stephan Rasp, Fiaz Ahmed, Paul A. O’Gorman, J. David Neelin, Nicholas J. Lutsko, Michael Pritchard, “Climate-Invariant Machine Learning”, Science Advances, 2024. [full text PDF]