Charlie Wood of Quantamagazine published in 2022 an article that highlighted the 2017 research work of Roger Guimerà and Marta Sales-Pardo, who discovered a cause of cell division – the process that drives the growth of life – using an unpublished and novel tool, a digital assistant they called a “machine scientist”. The method quickly gained acceptance, and Sales-Pardo & Guimerà are among a handful of researchers developing the latest generation of tools, known as symbolic regression. A description of the key elements of the tool can be found in Guimera et al. (2020).
In general, symbolic regression is a type of machine learning that can identify mathematical relationships between variables in data sets using Bayesian probability theory. It has been used to discover new equations that describe physical phenomena, such as the movement of fluids or the behavior of materials under stress. Researchers supporting the expansion of these methods say we’re on the cusp of “GoPro physics”, where a camera can point at an event and an algorithm can identify the underlying physical equation.
According to Wood’s article, machine scientists are being used in fields such as biology, chemistry and materials science to make new discoveries and accelerate scientific progress. For example, a team led by scientists at London-based artificial intelligence company DeepMind has developed a machine learning model that suggests the properties of a molecule by predicting the distribution of electrons within it.
If you are interested in the state of the art related to these approaches Liu et al. (2023) recently published the article “Data, measurement and empirical methods in the science of science”, which includes the Guimerà and Sales-Pardo experience. The publication is available in:
https://www.nature.com/articles/s41562-023-01562-4
If you are interested in Guimerà et al. (2022) “machine scientist” you can follow this link:
https://www.science.org/doi/full/10.1126/sciadv.aav6971
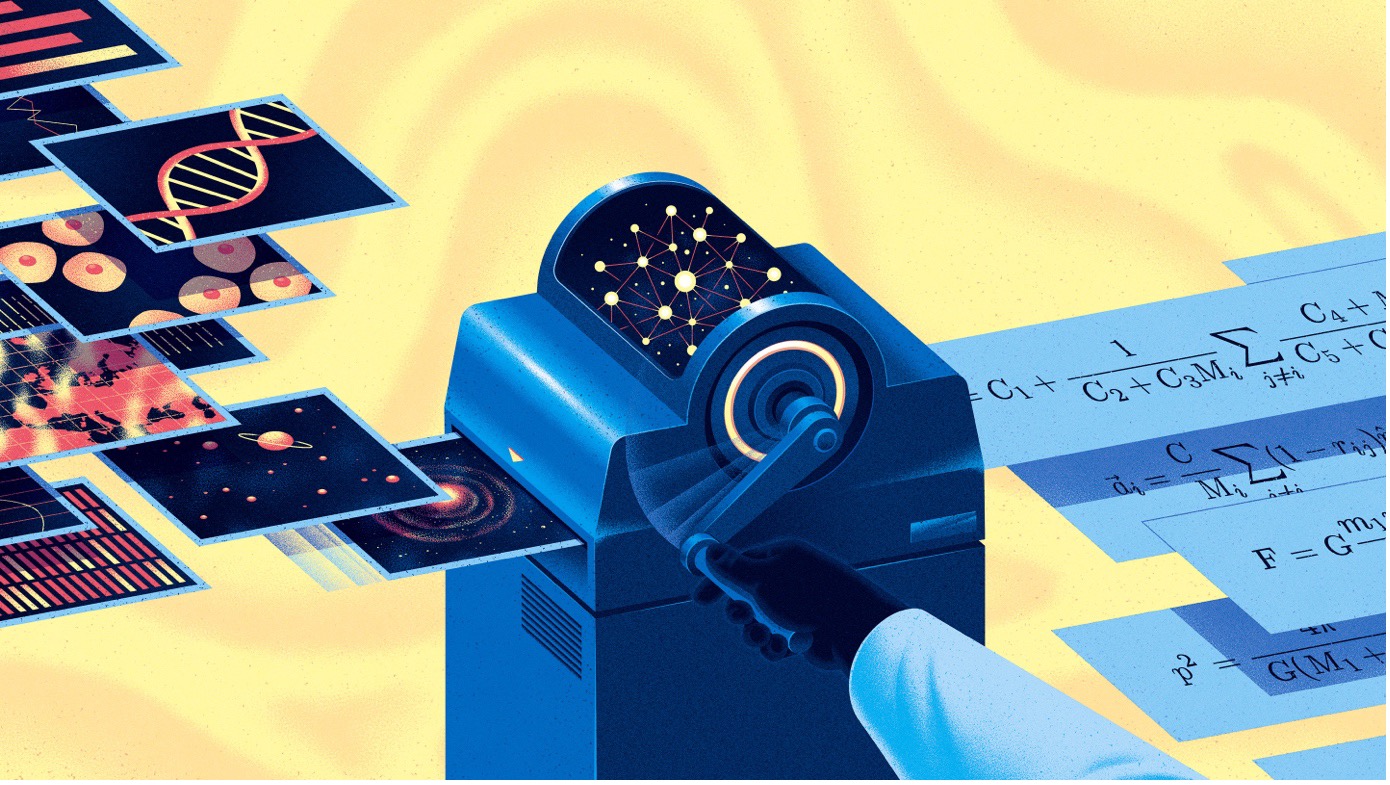
Image source: https://www.quantamagazine.org/