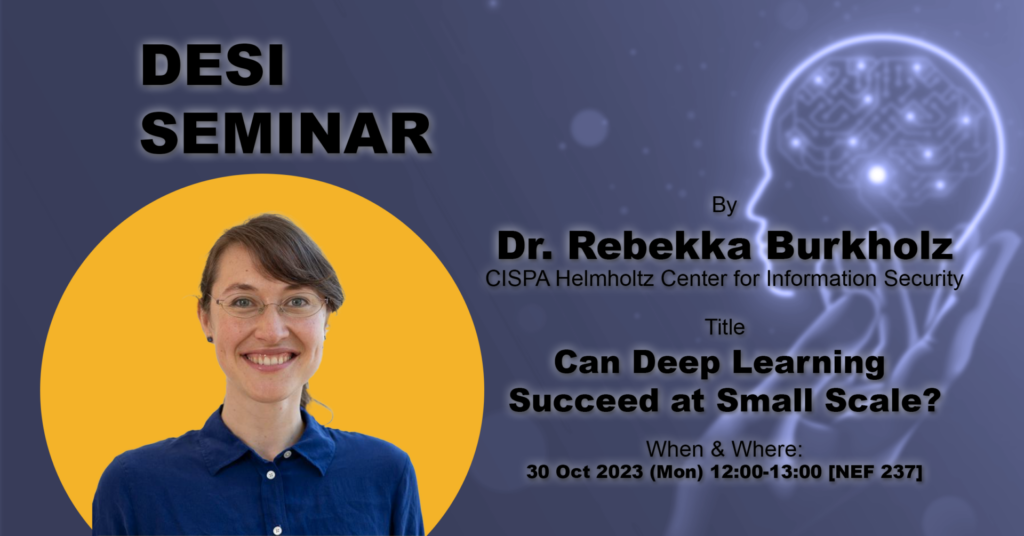
Dear all,
We would like to invite you to DESI seminar by Dr. Rebekka Birkholz [CISPA, Saarbrücken]
When & Where: 30 Oct 2023 (Mon) @ 12:00-13:00 [Internef 237]
Title: Can Deep Learning Succeed at Small Scale?
Abstract:
Deep learning continues to achieve impressive breakthroughs across disciplines but relies on increasingly large neural network models that are trained on massive data sets. Their development inflicts costs that are only affordable by a few labs and prevent global participation in the creation of related technologies. In this talk, we will ask the question if it really has to be like this and discuss some of the major challenges that limit the success of deep learning on smaller scales. We will give three examples of complimentary approaches that could help us address the underlying issues: (i) early neural network sparsification, (ii) the integration of useful inductive bias in the design of problem specific neural network architectures, and (iii) the improvement of training from scratch in the context of graph neural networks.
Bio:
Rebekka Burkholz is a tenure-track faculty member at the CISPA Helmholtz Center for Information Security in Saarbrücken, where she was recently awarded an ERC Starting Grant for her proposal on sparse machine learning.
Before joining CISPA in 2021, she spent two exciting years as a PostDoc in John Quackenbush’s lab at the Biostatistics Department of the Harvard T.H. Chan School of Public Health. Previously, she explored the intersection of machine learning and complex network science with biomedical applications as a PostDoc with Joachim Buhmann at the Institute for Machine Learning and at the Chair of Systems Design with Frank Schweitzer. Her PhD thesis on systemic risk at the ETH Risk Center won the Zurich Dissertation Prize and her work on international maize trade received the CSF Best Contribution Award. She studied Mathematics and Physics at TU Darmstadt.